Data Science Across Industries: Driving Innovation and Growth
February 14, 2025
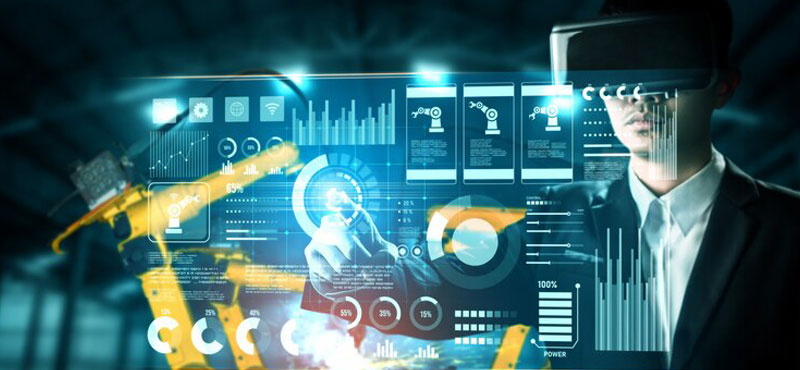
Data science can be described as a key growth engine within the context of today’s or the new digital economy. Companies use big data analytics to find patterns in extensive data sets to make business choices. Not only that, but they also help improve healthcare treatments, preserve financial stability, and many more areas where companies use raw data to find meaningful insights. This article discusses how various industries leverage data science to shape the future of technology, business, and everyone’s life.
Data Science in Healthcare
The healthcare industry has continued to expand with the aid of data science in the early diagnosis of diseases, individual treatment, and efficiency improvements. Data scientists work with large sets of medical information to help patients advance their medication experience and optimize the outcomes of managing a medical institution.
-
Diagnostic Applications: Innovative algorithms are used to diagnose dangerous diseases like cancer and diabetes early, enhancing patients' chances of survival. They also help raise awareness of high-risk patients so that measures can be taken to decrease the probability of their condition deteriorating.
-
Personalized Treatment: Depending on the patient’s genetic and medical state, a different treatment plan is recommended to minimize the side effects of trace elements and increase the effectiveness of treatment.
-
Drug Discovery & Development: Deep learning helps rapidly identify and develop drugs in various projects, assessing various molecular interactions and making it easier for companies to bring new medicines to market. For instance, deep learning models today have reduced drug development timelines by about 50%.
-
Real-time Health Monitoring: Mobile and IoT-based gadgets acquire constant patient health data, allowing them to be monitored continuously. This is very useful in quickly identifying conditions such as heart attacks or strokes.
Data Science in Finance & Banking
Data science plays a significant role in banking and financial organizations' implementation of robust security mechanisms and in making wiser decisions for the organization and customers. Data scientists help financial firms analyze vast volumes of structured and unstructured data to identify fraud cases, control risks, and tailor the products to the clients. Advanced machine learning models assist institutions in combatting competitors within the highly dynamic market.
Some of the primary uses of data science in finance and banking include:
-
Fraud Detection: Using machine learning, it is possible to detect real-time transaction indices and determine whether a transaction is fraudulent. Advanced autonomic faking detection systems do not generate many false alarms while delivering high outcomes.
-
Risk Evaluation: Credit scoring models evaluate the borrowers' risk level to minimize bad loans. Using historical data, institutions reduce risks associated with default.
-
Algorithmic Trading: High-frequency trading platforms apply analytics on the market trends and purchase or sell securities at the most advantageous time. This is because AI-focused strategies minimize intervention, and at the same time, the returns are optimized.
-
Customer Segmentation: It is a strategy that financial institutions use to gauge clients' behavior, income, or other financial needs. Individualized banking services and customized marketing solutions enhance consumers’ satisfaction.
Data Science in Retail & E-commerce
Data science is crucial in retail and e-commerce. It maximizes customer satisfaction, improves store inventory, and increases sales. Organizations can make the right decisions in an ever-changing marketplace just by analyzing large amounts of consumer data.
-
Dynamic Pricing Strategies: Retailers use data science to analyze customer behavior, competitor pricing, and market demand to adjust prices in real time. Various machine learning approaches enhance price elasticity prediction for maximum profit and customer satisfaction.
-
Personalized Recommendations: Websites that use artificial intelligence to review browsing history, check user purchases, and suggest similar products increase the conversion ratio and retain clients more effectively.
-
Inventory Management: Past experiences, future seasons, and even the overall economy determine the number of pieces that should be produced in any given period. This helps prevent excessive procurement and minimizes unsold inventories, which usually result in significant losses.
-
Customer Sentiment Analysis: Consumer reviews, feedback on social networking sites, and other opinions are used to determine customer satisfaction levels and trends. This aids in improving product portfolios and marketing approaches within the organization.
Data Science in Manufacturing & Supply Chain
Manufacturing and supply chain companies have adopted data science to increase productivity, cut costs, and improve product quality. AI and machine learning will be used for predictive analysis, which will help businesses implement a specific action plan to avoid unnecessary breakdowns and downtime costs.
-
Predictive Maintenance: The application of data sensors and algorithms makes it easier for manufacturers to foresee equipment failure in advance. It eliminates cases of sudden breakdowns and increases the utilization of products, hence increasing the useful life of machinery.
-
Demand Forecasting & Production Planning: Data scientists use sales data, market trend analysis, and other factors, such as weather conditions or geopolitical issues, to recommend the right time and quantity to produce and avoid unsold or produced products without a market.
-
Supply chain: Real-time analytics help optimize supplier, transport, and warehouse management. New models enable the company to reduce delivery time and overall expenses.
-
Quality Assurance & Quality Check: The advanced vision systems also help in quality assurance and checks, identifying defects and reducing the need for the actual removal of defective products. It increases production rate and, at the same time, does not compromise the set production standards.
Owing to the more popular and developed data science applications, manufacturing firms are experiencing better levels of automation, resource utilization, and environmentally friendly production, enhancing their profitability and global competitiveness.
Data Science in Entertainment & Media
Data science has a decisive role in the entertainment and media industry division as it aids in producing, promoting, and consuming content. Vast amounts of information allow a company to predict customers’ further behavior and offer them more engaging content. Data Scientists have done this in the following way:
-
Content Recommendation: Algorithms analyze viewing patterns, allowing tailored content suggestions that increase user retention. This improves engagement by providing interesting material that is of interest.
-
Audience Analysis: Detailing the audience’s demographics, affinities, and behaviors makes it easier for creators and marketers to create the right content and market it adequately.
-
Social Media Analysis: Sentiment analysis assesses the overall tone of social media interactions. Customers’ reviews and feedback determine the general direction of marketing and content.
-
AI-created content: Data science helps with script writing and editing, among other things, to increase production efficiency and reduce costs.
The Future of Data Science Across Industries
The practice of data science is being incorporated across all sectors, and newer and more complex application areas are expected in the future. Businesses are still exploring new horizons of data utilization through various technologies like artificial intelligence and machine learning. This continuous advancement opens up opportunities to optimize business processes, apply innovations to customer services, and innovate in aspects that were not achievable earlier.
Here are the future trends in data science:
-
Intelligent automation: A culture of intelligent automated concurrent learning will exist across several sectors of the economy, especially the financial, manufacturing, and logistic industries.
-
Real-time big data processing: New technologies like cloud computing and IoT will allow industries to make real-time decisions in many sectors, such as healthcare, retail, transportation, etc.
-
Ethical and Legal Concerns: Integrating AI and ML into industries would entail ethical and legal questions regarding privacy, equality, and legal and user transparency.
Conclusion
Data science is becoming increasingly popular globally due to its impact on different operations, innovations, and even decision-making. Since the need for data scientists is rising, the world economy depends increasingly on data science for a competitive advantage. AI, machine learning, and analytics have become integral to various industries, making data science a key to their further growth and sustainability.