Ensuring Trustworthiness: The Crucial Role of Data Science Ethics
August 09, 2024
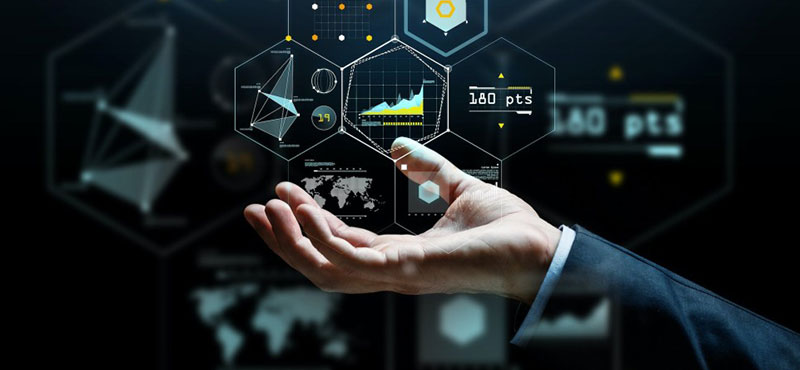
With data science becoming the backbone of innovations in the modern world, it is essential to consider the ethical aspects of data. Data science ethics are principles that govern proper and appropriate use of data, and that seek to respect privacy, establish non-discrimination, and increase the management of information. Data science is gradually becoming central to decision-making in society; thus, it is crucial to appreciate and apply ethical principles in protecting data integrity. This article aims to present the key issues and approaches in data science ethics.
The Fundamentals of Data Science Ethics
Data science ethics refers to the set of rules that help to better understand the usage of data and algorithms. They serve to ensure that data science applied in practices is equipped with qualities such as transparency, accountability, and fairness. Several ethical standards should be followed to keep trust and extend the use of data in decision-making. Some of the main considerations are privacy, non-biasedness, data subject consent, etc. Thus, integrating ethical aspects into data science initiatives ensures that adverse impacts are mitigated, consumers’ confidence is established, and data and innovative solutions are utilized appropriately.
Common Ethical Issues in Data Science
It is critically important to address ethical issues in data science, as the ability to process large amounts of data may significantly influence people’s lives and societies. Solving these problems implies deep recognition of the processes of data gathering and analysis, as well as their responsible and fair application.
Common Ethical Issues in Data Science:
-
Privacy Concerns: Privacy is a major concern that stems from the collection and application of personal data. Data scientists merely work and analyze big data without fully capturing people’s privacy while at the same time, they can work to find something meaningful in the data. Anonymization of the information and imposing strict access measures are effective ways of protecting personal data.
-
Bias and Discrimination: One limitation of algorithms is their ability to encode biases that may exist within the dataset used for training. This can result in prejudiced decision-making when it comes to issues like hiring, credit extensions, or even policing. To control bias, it is crucial to be more careful with data samples used as well as with algorithms implemented and their further monitoring to detect and correct any signs of bias.
-
Transparency and Explainability: While decisions about different aspects of life are informed by data, the algorithms involved are not always transparent. This often leads to failure, whereby stakeholders remain unaware of how decisions are made or how their data affects the final decision-making. Transparency is about making algorithms understandable and providing effective explanations of how information is processed to come up with specific outcomes.
-
Informed Consent: The sustainability of choices and how individuals consent to data collection and use is very important but challenging. Related questions emerge when consent documents are vaguely and poorly communicated; leaving data subjects uncertain about how their collected data will be used. The consent process should enable individuals to make choices about how to control their data.
Principles of Data Ethics
In data science ethics, it is critical to follow certain principles to use data in a righteous and just way. These principles help practitioners and organizations work in the various realms of data gathering, interpretation, and application, while ensuring that people’s rights are protected.
1. Fairness
-
Objective: Avoid data discrimination, which means that one should not practice certain data practices and make decisions that would benefit one group over the other.
-
Implementation: Use algorithms and models that are non-discriminatory and fair to all genders, ethnicities, and other categories of people.
-
Example: Changing algorithms so that they don’t reinforce past discrimination in things like hiring or loan approvals.
2. Accountability
-
Objective: Make people and companies responsible for the moral impact of their data science actions.
-
Implementation: Define responsibilities for ethical decision-making and make sure that every decision made is documented as being made by somebody.
-
Example: Recording actions that are implemented concerning the data and ensuring that everyone involved understands their roles.
3. Transparency
-
Objective: Ensure that the information given is simple and easy to comprehend when it comes to the collection and analysis of data and the decision-making processes.
-
Implementation: Reveal the data sources and the methods applied to derive insights, as well as the implications of data-driven decisions on the stakeholders.
-
Example: Releasing the algorithms applied in credit scoring so that consumers can know how the decisions are made.
4. Privacy
-
Objective: Prevent the exposure of individuals’ personal information to unauthorized persons or entities.
-
Implementation: Put strong data protection mechanisms in place and make sure the organization adheres to the existing privacy laws (e.g., GDPR, CCPA).
-
Example: Alteration of data so that the data controller can no longer identify the data subject without the data subject’s consent.
5. Responsibility
-
Objective: Understand and appreciate the ethical concerns associated with data science practice and adhere to ethical considerations when making choices.
-
Implementation: Develop an ethical mindset across the organization and make ethical considerations part of people’s thinking and actions.
-
Example: Education of employees regarding the right and wrong way of data management and reporting of ethical issues.
These principles form the basis for the ethical practice of data science, thereby helping data scientists and the organizations to use data appropriately while being conscious of the potential impacts and outcomes of the data use. Thus, by incorporating these principles into daily practice, key players in the field can reduce the risks, promote diversity, and adhere to the best ethical practices in the field of data science.
Strategies for Implementing Data Science Ethics
Effective data science ethics cannot be achieved without a proactive approach that ensures ethical considerations are incorporated at various stages of the project. Here are key strategies to ensure ethical practices in data science:
-
Ethical Guidelines and Frameworks: Develop clear ethical standards that include principles such as openness, non-bias, and accountability. These frameworks should be adjusted to respond to certain ethical problems that are typical for the organization and industry.
-
Bias Mitigation Techniques: Utilize more intricate strategies for identifying and reducing the bias in data and machine learning algorithms. This includes checking datasets for potential demographic or other forms of bias and modifying the algorithms to avoid unfair results.
-
Transparent Practices: Promote clear communication by defining data-gathering procedures, model development techniques, and decision rules as transparently as possible. This fosters credibility and enables one to demonstrate that they are answerable to others.
-
Stakeholder Engagement: Engage the people who are affected by the data science project, ethicists, and the community in which it will be implemented. Thus, their input can help identify possible ethical concerns and prevent certain initiatives from conflicting with the general moral standards.
These strategies help to prevent ethical risks and strengthen the accountability and social relevance of data science projects and developments in the tech world.
Case Studies: Examples of Ethical Data Science Practices
In the data science ethics, real-world examples illustrate both successful implementations and challenges faced in ethical practices:
-
Healthcare Project Success: A healthcare organization has very strict ethical principles in its data science projects, particularly related to patients’ information and data protection. Through this, they ensured that all activities related to data were properly regulated and that every patient’s data was used with their permission. Activities such as surveys and audits were useful in keeping ethical practices in check at all times.
-
Social Media Platform Challenges: A big social media site came under fire for ethical violations in data management. They unintentionally reinforced prejudices, thus contributing to unfair results in content delivery and advertising. This case demonstrates why it is essential to have proper measures to address the bias and ensure the openness of the algorithm’s creation process. This case highlighted the need for regular ethical checks and preventive measures in combating biases in technological applications dependent on data.
These cases illustrate that data science ethics are not always clear-cut and that having ethical guidelines and being transparent can help build trust and prevent misuse of data and bias.
Conclusion
Data science ethics is important in promoting proper innovation as a way of improving lives in society. Thus, following the principles of fairness, transparency, and accountability, organizations can gain trust and credibility. It is therefore important for data science professionals and any other stakeholders to consider ethical issues to avoid adverse effects and promote a healthy society powered by ethical data analysis.