How AI transforms data science and drives smarter decisions?
December 20, 2024
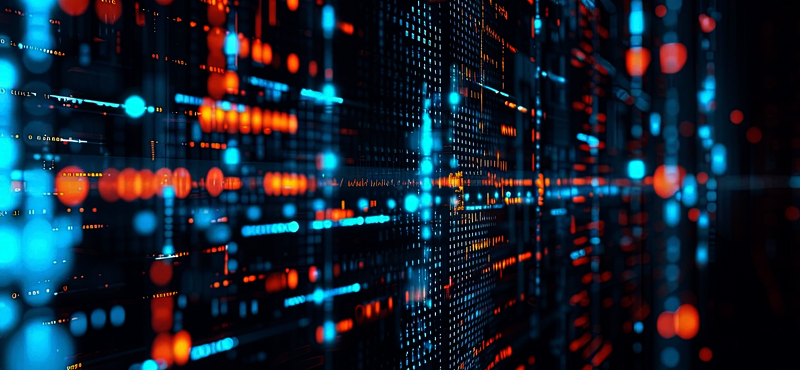
AI has opened new opportunities by integrating with data science. It has become indispensable for modern data-driven strategies by automating complex tasks, improving predictive accuracy, and uncovering hidden insights. AI allows businesses and professionals to collaborate and solve challenges with precision and speed, resulting in innovation across all industries. The application of AI across various fields of data science is vital for success in today’s competitive landscape.
The Evolution of AI in Data Science
AI in Data Science has changed how we process, analyze, and interpret our data. Data science started as a domain of basic statistical methods and manual data analysis. However, the development of machine learning (ML) and deep learning (DL) has made AI enable data scientists to automatically process large datasets with much greater precision.
Significant milestones in this evolution include:
-
From traditional algorithms to intelligent models: The early AI was based on a rule-based algorithm and today AI is using a complex model such as a neural network with other data, allowing for more dynamic and nuanced data analysis.
-
Integration with big data technologie: With the increasing volume of the data, AI in data science started to work as a spear that has helped in handling and processing big data and made it easy to derive actionable insights.
-
The rise of automation: The automation of routine tasks such as data cleaning, feature selection, and model tuning has changed workflows, allowing data scientists to spend more of their time-solving higher-level problems.
Key Applications of AI in Data Science
Data processing and data analysis are being transformed by AI in every industry. AI's integration of machine learning algorithms and deep learning models improves the different aspects of data science. Some important applications include:
-
Data Processing and Cleanin: AI enhances data visualization by identifying patterns and presenting them in intuitive formats. AI with techniques such as natural language processing cleans unstructured data and makes this data more accurate to analyze.
-
Predictive Analytics: Futuristic trends can be predicted using historical data by AI models. Such models are useful in different industries, including finance for stock market predictions, healthcare for disease forecasting as well as marketing for customer behavior prediction, etc.
-
Data Visualization: Data visualization is improved by AI in the form of identifying patterns and presenting them in forms that are easy to understand. Dynamic, interactive visualizations made possible by advanced algorithms enable complex data to be easier to interpret and help decision-makers take action.
-
Anomaly Detection: Anomalies or outliers can be detected automatically in large datasets through AI, which is critical for fraud detection, network security, and monitoring industrial systems. They can learn from data patterns and then flag deviations even more efficiently than with manual methods.
AI-Driven Algorithms in Data Science
Data Science is powered by AI algorithms that allows for sophisticated analysis and there is efficiency in making decisions. Here's a breakdown of how different AI-driven techniques are used in data science:
-
Supervised learnin: In supervised learning, a labeled dataset is input to the algorithm, which ‘learns’ to predict outputs based on known inputs. This is used really widely for classification and regression tasks, being how data scientists develop predictions with high accuracy.
-
Unsupervised learning: These algorithms do not work on labeled data and instead teach humans to find hidden patterns or groups within the dataset. Clustering data points and reducing the dimensionality of datasets are important tasks, especially used in exploratory data analysis.
-
Neural networks and deep learning: Deep learning models simulate human brain functioning and their capability in dealing with big and complex data sets is unrealized. For the task of image recognition, natural language processing, time series forecasting, these models will be crucial.
-
Reinforcement learning: It allows the machines to learn through trial and error. When continuous learning from the environment is required, as in decision-making tasks like optimizing business operations, self-driving cars, or robotic systems, it is particularly useful.
Enhancing Data Collection and Real-Time Analysis
AI significantly enhances data collection and real-time analysis, revolutionizing how businesses and data scientists interact with vast datasets:
-
Automating data gathering: AI can automatically collect data from many sources instead of manually. It helps speed and accuracy in the data acquisition process.
-
Real-time processing: This allows businesses to have the ability to process incoming data as soon as it is being collected thus it can be analyzed in time and decisions can be implemented in real time. In environments where real-time responses are critical, such as stock trading or live customer support, this is particularly handy.
-
Improved data accuracy: Data collection is largely assisted by AI to reduce human error in the process. Using intelligent sensors and algorithms, AI is better at taking better data from various sources, rather than being more accurate.
-
Integration of multiple data streams: The data can be seamlessly integrated from multiple sources including sensors, transactional systems, and user interactions, via AI. It gives companies the ability to get a perspective of what’s taking place across all the channels involved.
AI for Enhancing Accuracy and Reducing Bias
Data-driven decisions are improved by the use of AI to mitigate the risk of bias. It is based on sophisticated algorithms so that AI naturally processes large amounts of data with greater precision than mere humans. Keyways AI enhances accuracy and reduces bias:
-
Increased accuracy of predictions: AI models can work with large datasets and identify complex patterns to give more accurate predictions. These models can learn from data continuously, making sure they are highly capable of delivering highly reliable results.
-
Minimizing human errors: Data analysis using AI reduces the possibility of making human mistakes. Automation of data cleaning and model optimization prevents inaccuracies that typically arise from manual interventions.
-
Bias detection and correction: Tools for AI can identify bias in the data, especially because of racial, gender, or socioeconomic biases in the dataset. The models can creatively develop algorithms to minimize the tendency to discriminate and therefore have better outcomes with less bias.
-
Improved decision-making: It removes subjective judgments from the decision-making process aided by AI. This avoids subjectivity as to what choices are made — only facts can be used in the analysis.
Future Trends: The Next Frontier for AI in Data Science
AI in data science is on the verge of a revolution that will reshape data analysis and utilization in ways that have never been seen before — with unprecedented efficiency and creativity.
Transformative advances in emerging trends allow data scientists to meet the most difficult problems with ever higher degree of precision.
-
Autonomous Machine Learning (AutoML: These systems are beginning to become AI systems that can take over the entire machine learning process, from model selection to hyperparameter tuning, greatly decreasing the amount of human intervention.
-
Advanced Natural Language Processing (NLP): In future applications, text and speech unstructured data can be understood better by AI which makes it more essential in understanding human inputs.
-
Real-Time Data Analytics: Advances in AI will deliver seamlessly integrated real-time insights, and industries like healthcare and finance will be able to make swift data-driven right decisions like never before with unprecedented accuracy and efficiency.
-
Edge AI Integration: Data processing closer to source devices, with lower latency and more security, is enabled by AI-driven analytics at the edge.
Conclusion
Data science with AI has been a game changer for data processing, analyzing, and usage where precision, speed, and automation are unmatched. It is reshaping the boundaries of what data science can do by enhancing predictive analytics, reducing bias, and providing real-time insight. Cutting-edge AI techniques are integrated to keep businesses competitive in a data world. The more we continue to see AI mature, the more it will have its synergy with data science and unlock even greater innovation and transformative possibilities.